Introduction
The term data analytics (Data analytics in finance) broadly references the processes of collecting and assessing various sets of information. Multiple techniques can be employed to extract data and analyze patterns and trends that contribute to practical insight, inform further research, or decide on effective business strategies. As the process of analyzing raw data to find trends and answer questions, the definition of data analytics captures its broad scope of the field. However, it includes many techniques with many different goals.
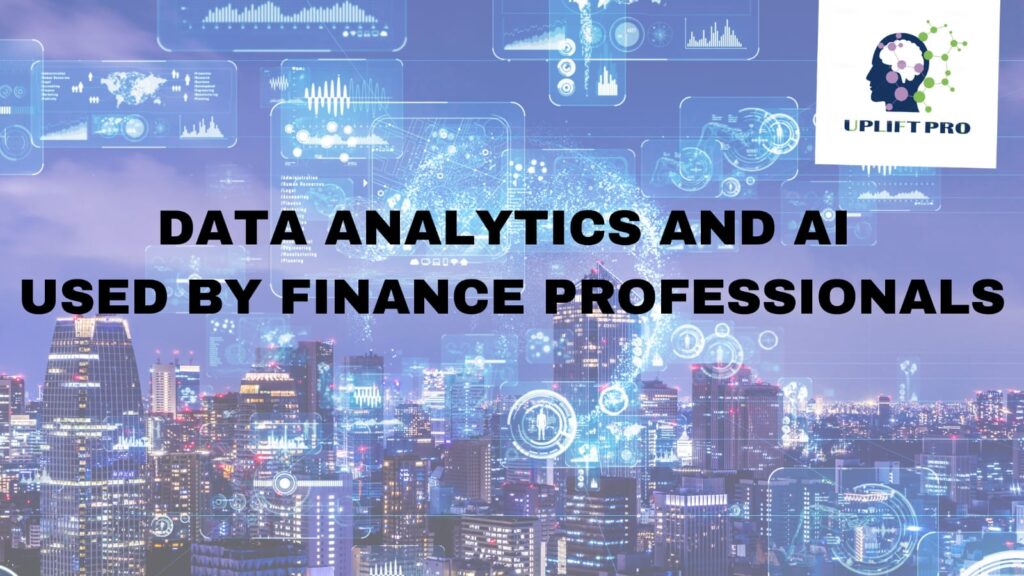
The data analytics process has some components that can help a variety of initiatives. By combining these components, a successful data analytics initiative will provide a clear picture of where you are, where you have been and where you should go.
A. Descriptive analytics
Generally, this process begins with descriptive analytics. This is the process of describing historical trends in data. Descriptive analytics aims to answer the question “what happened?” This often involves measuring traditional indicators such as return on investment (ROI). The indicators used will be different for each industry. Descriptive analytics does not make predictions or directly inform decisions. It focuses on summarizing data in a meaningful and descriptive way.
B. Advanced analytics & Machine learning
The next essential part of data analytics is advanced analytics. This part of data science takes advantage of advanced tools to extract data, make predictions and discover trends. These tools include classical statistics as well as machine learning.
Machine learning technologies such as neural networks, natural language processing, sentiment analysis and more enable advanced analytics. This information provides new insight from data. Advanced analytics addresses “what if?” questions. The availability of machine learning techniques, massive data sets, and cheap computing power has enabled the use of these techniques in many industries.
The collection of big data sets is instrumental in enabling these techniques. Big data analytics enables businesses to draw meaningful conclusions from complex and varied data sources, which has been made possible by advances in parallel processing and cheap computational power.
C. Data analytics in finance
Data analytics in finance is growing in importance. Globally, an increasing number of businesses are using data analytics to improve internal operations. They also rely on data analytics to help them understand their customers on a deeper level. This allows organizational leaders to make informed decisions that promote better business outcomes.
Data analysis can benefit organizations across all industries. This is especially true of financial institutions, which often have a sea of raw data to sift through. Used correctly, financial data, such as purchasing behavior, along with credit card data, can be invaluable to these companies.
Five Key Types of Big Data Analytics Every Business Analyst Should Know
· Prescriptive Analytics.
· Diagnostic Analytics.
· Descriptive Analytics.
· Predictive Analytics.
· Cyber Analytics.
Technical Skills Required for Data Analysts
· Data Visualization. – As the term suggests, data visualization is a person’s ability to present data findings via graphics or other illustrations
· Data Cleaning
· MATLAB.
· R.
· Python.
· SQL and NoSQL.
· Machine Learning.
· Linear Algebra and Calculus.
The Importance of Data Analytics in Finance
The coronavirus pandemic has caused a tremendous amount of uncertainty in the finance sector.
“The lighthouse in this uncertainty is the ability to use advanced data analytics to better manage finances. When a company is able to masterfully forecast cash flow and execute on its strategic financial visions, it is empowered to serve its market and clients for decades to come.”
said Bassem Hamdy, author of The Importance of Data analytics in finance
Hamdy also explained that implementing a financial strategy starts with possessing an understanding of the true financial position of a company. This entails having the ability to answer questions using operational and financial data, not gut alone. This is where a financial data analytics professional comes in.
Data Analytics: A Revolution in Finance Industry
According to an article by Softweb Solutions, data analytics is revolutionizing the finance industry. One way it is accomplishing this is by reducing the component of human error from daily financial transactions.
The article lists other reasons why data analytics in finance has transformed the finance sector:
1. Data analytics enables finance executives to turn structured or unstructured data into insights that promote better decision making.
2. Data analytics in finance helps finance teams gather the information needed to gain a clear view of key performance indicators (KPIs). Examples include revenue generated, net income, payroll costs, etc.
3. Data analytics allows finance teams to scrutinize and comprehend vital metrics, and detect fraud in revenue turnover. This is helpful since financial services experienced a huge increase in digital fraud activity in 2020.
4. Additionally, big data has improved the way stock markets work and has upgraded investment-related decision making
The Role of a Finance Data Analyst
Finance data analysts are professionals who help financial institutions utilize data to make high-quality business decisions. One of their primary roles is examining financial records. They do this for the purpose of preparing in-depth reports for a financial organization.
Financial analysts often work with key organizational leaders, such as chief financial officers (CFOs). They help these professionals ensure the company makes sense of its raw data and benefits from it. The best candidates for a finance data analyst role are often junior analysts that support business functions. These functions include marketing, finance or operations roles. However, these individuals are usually asked to work closely with data to interpret and communicate what they find in the data.
Finance data analysts often are knowledgeable of and proficient in skills related to the following topics:
· Data mining
· Financial analytics
· Understanding business models
· Financial forecasting
· Creating financial models
· Risk management
· Big data analytics
· Advanced analytics
· Data management
· Predictive analytics
· Microsoft Excel
· Algorithms and algorithmic trading
· Python
· Automation
· Data science
· Business intelligence
· Machine learning
· Artificial intelligence
· Real-time data flows
Data Analytics for Business
Businesses may collect and analyze historical or trending data. The type of data collected depends on the intent for its use. Data may also be collected directly from customers, site visitors, or purchased from third parties. This data ranges widely in terms of content.
Common interests for data often include information on customer demographics, interests, behaviors, and numerous other factors a business determines relevant. It is essential to realize that data analytics are increasingly becoming more necessary across industries.
Common associations of data analytics with marketing and internet commerce are a shortsighted mistake. Broad diversity of businesses and industries can gain competitive advantages from data analysis insights, which is now more accessible than ever before. Companies can better understand their customer’s profiles, habits, and interests. This vital data can be used to inform decisions that maximize profits and customize user or customer experiences to increase efficiency and loyalty.
Implementing Data Analytics for Business
A few core tenets will assist in optimizing the results a business produces from its data analysis. Figuring out what data to collect is a first step for many companies. Data can be derived from social media, GPS, and other like technology, transaction information, and various other sources. Evaluating the accuracy and pertinence of data is also critical. Data can be collected and analyzed, but this does not guarantee its accuracy or applicability to a business’s goals or strategy. Assessing how the data was collected can be a practical first step in checking for flaws, inaccuracies, or relevance. Finally, data should be conceptualized for actionable steps.
A business’s data analytics should present the vast and obscure data sets into informational representations that are accessibly understood. These representations must then be applied to actions that can elevate chances for success. A company may want to increase security, drive traffic to its website, refine customer service, or directly build revenue. Learning to assess and implement data analytics can require significant effort. But expertly trained and highly resourced support is available to assist with these complex and critical endeavors.
The Future Role of Data Analytics in the Finance Industry
The future role of Data analytics in finance is secure as data analysis is critical to the success of financial institutions. After all, as the finance sector continues to digitize, there will be more raw data for organizational leaders to interpret. Data analytics will help them make use of the data. Amazingly, just 0.5% of businesses make use of their data, according to Data and Analytics in Financial Services. Those who practice financial data analysis can help organizations make the most of the data they collect.
Summary
In the current economic condition in the post pandemic era, data analytics allows businesses and more specifically the finance industries to modify their processes based on these learnings to make better decisions. This could mean figuring what new products to bring to market, developing strategies to retain valuable customers, or evaluating the effectiveness of new medical treatments. newer dimensions now due to shift from conventional, paper-based manual methods to more complex, Internet-based techniques.
The needs of businesspersons from yesteryears were different than those of today. Because the bulk of business transactions or at least some part leading to deals occurs online. Analysis of data, learning from its results and utilizing them can help every business cut losses while boosting profits. E-commerce is here to stay and grow exponentially. In such scenarios, only data and analytics can help a company flourish, if put to proper use.
In fact, businesses that fail to utilize data and analytics are sounding their own death knell. Accordingly, it is the need of the hour that finance professionals should cope with the changing scenario by embracing the tools and techniques of data analytics and amalgamate with their finance/accounting skill for a brighter future ahead.
Choose the best US CPA, US CMA, US CIA, Data Analytics in Finance, SOX Compliance Certification institute in India, Africa, and Middle East – Uplift Pro
Uplift Pro is one of the top training institutes for the US CMA, US CPA US course, US CIA course, Data Analytics in Finance, SOX Compliance Certification, Enrolled Agent course in India, Africa, and Middle East. Uplift Pro is also an Indian partner of GLEIM, US and an IMA US authorized CMA US study center.
Our team consists of seasoned professionals and entrepreneurs from IIEST, IITs, London Business School, and ULCA who have decided to provide a strong backup to young ambitious students and professionals to reach their desired career destinations in an organized way.
Some of the exclusive features include –
A. Authorized partner of IMA and Gleim in India
B. High pass rate of 85 %
C. Live online classes ensuring that the regular office working hours is least impacted
D. 1:1 personal support from our 30 plus years of experienced US CMA and CPA US course certified faculties
E. Affordable course fees
Request for Live Demo class / contact at +91-8787088850 to book your seats now.
kamagra livraison 24h: kamagra pas cher – Kamagra Oral Jelly pas cher